Introduction
Evaluating the greatest college coaches across various sports involves analyzing multiple factors such as total wins, win rate, championships, and player performance. This blog highlights the mathematical models used to rank college coaches in football, basketball, and women's hockey, focusing on fairness, objectivity, and adaptability to different time periods.
Key Methods Used
1. Analytic Hierarchy Process (AHP)
- Organizes indicators into a three-layer hierarchy, prioritizing features like total wins, number of championships, and coaching age.
- Weights are determined using subjective expert judgments and then applied to compute overall scores for coaches.
- Strength: Simple and intuitive; widely applicable to multi-criteria decision-making.
- Weakness: Relies heavily on subjective input, which can introduce bias.
2. Technique for Order of Preference by Similarity to Ideal Solution (TOPSIS)
- Ranks candidates based on their proximity to an ideal solution and distance from a negative solution.
- Incorporates AHP-derived weights but removes the need for a hierarchical structure, making it more streamlined.
- Strength: Provides clear rankings without requiring a strict hierarchy.
- Weakness: Still depends on subjectively determined weights.
3. Principal Component Analysis (PCA)
- Reduces dimensionality by combining correlated indicators into fewer principal components.
- Ensures objectivity by determining weights directly from data without subjective judgment.
- Strength: Generates unbiased, data-driven rankings.
- Weakness: Ignores the time horizon of coaching achievements.
4. Logistic Regression (Time-Revised Model)
- Adjusts rankings to account for the evolving popularity of sports over time.
- Uses a logistic growth curve to reflect the changing scale and influence of sports, ensuring fairness across different eras.
- Strength: Provides a balanced comparison between coaches from different time periods.
- Weakness: Requires detailed historical data, which may not always be available.
Conclusion
The combination of these models provides a robust and comprehensive framework for evaluating college coaches. By addressing biases, incorporating objective data analysis, and factoring in historical context, these models offer nuanced and equitable rankings. Future applications could include exploring new indicators or extending the models to other sports beyond the NCAA.
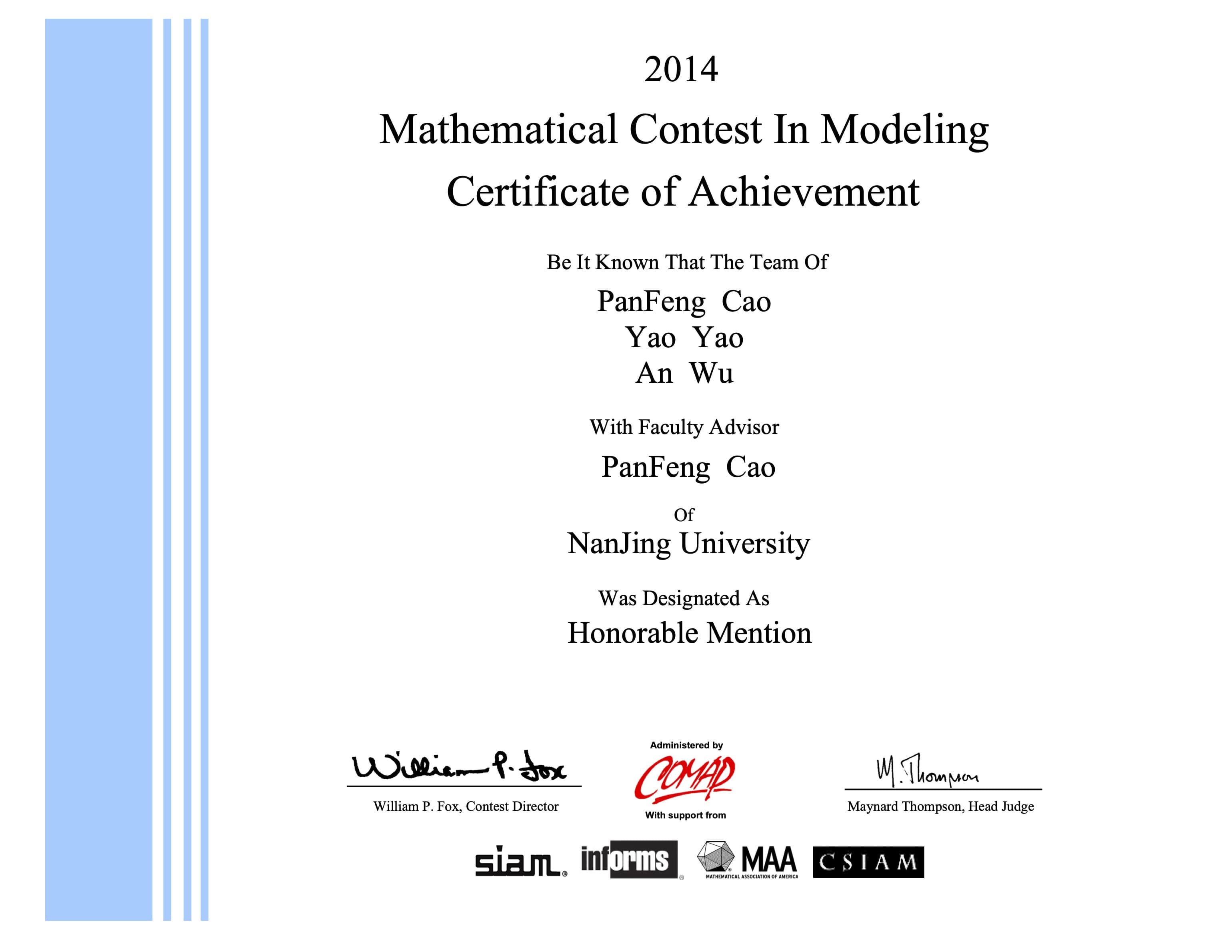